Dear members of the EU AI Alliance,
Virginia Dignum and myself are members of the High Level Expert Group on AI and co-rapporteurs of the section on Accomplishing Civil Society Engagement & Benefits of AI. This section is a part of Deliverable 2 of the High Level Expert Group on AI: Policy and Investment Recommendations for AI.
A prequisite for achieving beneficial impact of AI on society is knowledge of and insight in the actual broad societal impact of AI.
In order to determine and measure the societal impact of AI, a mechanism to continuously gain insight, measure and score this impact is necessary. At our latest meeting of a subgroup of members of High Level Expert Group we decided to request input on appropriate mechanisms to measure the broad societal impact of AI from the EU AI Alliance.
In short, we are looking for input on existing or new mechanisms that will help gain insight and measure the impact of AI on all area's of life that may affect our general well-being: labour, health, education, (in)equality, housing, wealth, poverty, social protection, privacy, safety, freedom etc. We know there are a large number of well-being metrics available as well as social impact scoreboards that might be of use, but we are looking for input as to how the use of AI impacts society in a positive as well as a negative or challenging manner.
- Kirjaudu sisään, jotta voit julkaista kommentteja
- Tunnisteet
- AI HLEG - Input request
Kommentit

Hi,
Measuring the impact of AI on society in such broad terms is something all would like to know, and especially governments. It is however far from trivial. One of the difficult things to measure is how much of the impact is due to AI and how much is due to other elements of a digital transformation, or even a culture or process change. I did some work on measuring the impact on big data on businesses, which encounters the same problems, yet much simpler. Maybe you can get some inspiration from it: https://data-speaks.luca-d3.com/2017/01/big-data-whats-economic-value-o…
There is also quite a lot of (research & pilot) work on Big Data and AI for Social Good, which is about measuring and helping achieve the UN's Sustainable Development Goals. There is a lot of privately held data (telcos, banks, retailers, utility companies, satellite companies) that could provide proxies for measuring societal impact of government measures (poverty, literacy, climate change, migration, peace, etc). Maybe this can give you ideas of how to measure the impact of AI on society.
http://unglobalpulse.org/sites/default/files/MobileDataforSocialGoodRep…;
https://www.slideshare.net/LUCA-D3/data-as-a-force-for-good
Again, it is a tough topic, but hope this helps a little bit.
Kind regards,
-- Richard
- Kirjaudu sisään, jotta voit julkaista kommentteja
In reply to Hi, by Richard Benjamins
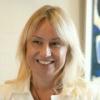
Thank you Richard.
- Kirjaudu sisään, jotta voit julkaista kommentteja
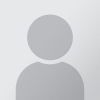
User account was deleted
- Kirjaudu sisään, jotta voit julkaista kommentteja
In reply to In the Commission, we by Anonymous (ei varmistettu)
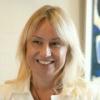
Thank you Bjorn!
- Kirjaudu sisään, jotta voit julkaista kommentteja

AI and Society
Measuring and monitoring the societal impact of AI
Nations that rise ahead in the AI revolution will be those that not only harness the advantages of AI, but also have an effective plan for managing the societal disruption that it will bring. (Strategic Competition in an Era of Artificial Intelligence)
I would like to start with some general considerations.
First, the societal impact of AI is not directly measurable, except maybe for a few relatively mundane aspects (investments, number of people working in AI-related fields, number of deployed AI, energy consumption of AI systems, etc); the most politically relevant forms and facets of this impact are to be inferred analytically. Some of the impact might very well be qualitative, subtle, like a change in the mechanism of influence of one societal dimension upon another. Last but not least, when assessing the impact we should step beyond the aggregate effects, otherwise we risk disregarding the distributional aspects of rewards and loses, the fact that AI produces both winners and victims.
Second, in order to measure impact, we have to decide what is the input. Thus, we have to agree on a definition / extension of AI, because this is definitely not an objective, homogeneous, undifferentiated class of existence. Fow example, do we include chatbots, smart contracts, routing software (Waze), and Google Translate? We should also keep in mind that the generally accepted view of what constitutes AI might change over time : once we reach the stage of semantic AI (capable of discerning the meaning of words, objects, and actions), will we still consider the old non-semantic face recognition systems, chatbots, or autonomous cars as intelligent?
Third, there are several sides / components of the input, which generate different forms and mechanisms of impact on society :
(1) Objective side (tools, explicit instructions, process knowledge)
(2) Semantic / ideological load, the meaning associated, the narratives that establish the background, role, and character of technology, its relationships with other societal facets and phenomena, notably politics. There is meaning given (bestowed by public figures and the intelligentsia), and meaning embedded (the values and ideals, dreams and fears, of the industries / organized groups that develop and implement AI, in which the technology is rooted). This sets the arena in which technology can manifest itself, and shapes the influence mechanisms.
(3) Interaction of "cultural areas", with different visions / perceptions / ideologies / approach regarding AI. The same culture will not be dominant and undisputed worldwide. e.g. EU focuses (for the moment) on ethics and legal aspects, while in China the development and deployment of AI is subordinated to the national rejuvenation project, aimed at "enhancing national power".
Let's look in turn at first two sides of the input. Both should be considered, in order to allow the direct examination of the interplay between objective and inter-subjective factors; the latter type of factors cannot be dismissed since public perceptions and preferences shape or restrict policy - like the anti-GMO movement, or Germany's decision to abandon nuclear power.
(1) In order to determine the characteristics and magnitude of the objective side of AI, we could (upon agreeing on a definition / extension) conduct a continuous, administrative census. This could be achieved by implementing legislation requiring the disclosure, and possibly registration, of every AI used. The data is collected by the public administration, in the same way it collects data on SME or vehicles. At a minimum, use of an AI or Automated Decision System should be clearly indicated on any document (including web pages) that communicates the decision (or any output of the decision-making process) to the affected person / institution / group. Such universal disclosure requirements should contribute to the building of public trust. Among the difficulties of this approach is the phenomenon of fauxtomation / "Potemkin AI", meaning that algorithms are given undue credit, and processes are misrepresented as AI to devalue the human labor that is actually doing the work.
(2) Let's start with a brief example of semantic load and its potential impact. If we perceive AI as inherently benign and trustworthy, we are more likely to embrace it (higher speed and scope of adoption), to be oblivious or at least permissive regarding inbuilt biases (allowing for the reproduction of inequality and discrimination structures), and to assume that those left behind will also get well in the end (resulting in less support for social security and reskilling policies and programs). If AI is seen as neutral, objectively inevitable, we might relinquish our societal right to shape and direct it (thus perceiving as illegitimate or at least useless the efforts to establish an AI ethics), disregard the stewardship and even entrepreneurial role of the state, and leave the initiative to Silicon Valley and venture capitalists.
The object of analysis here is the public discourse on AI (including, but not limited to, legal texts, articles, speeches, handbooks and other educational material, internal company documents, memoirs and interviews of key figures of technology, advertisements), collected e.g. by web scraping and document collection, and de/re-coded through content analysis and hermeneutics. This is arguably the most work-intensive part, but I would say it is crucial because it would illuminate the mechanisms of influence, and elucidate differences between cases that are similar on the objective component.
A special category are AI-related laws and regulations : they have a quasi-objective, reified nature (so they can be seen as a complement of the objective side of technology), but they are also the embodiment and balance of value systems and interests.
A few words now on the impact.
Technology is reflected at personal level in experiences and practices, as well as in attitudes, opinions, value systems, and worldviews. At the level of society, technology can impact the size of the workforce and its skill and demographic composition, labor and demographic mobility, forms of sociability and patterns of human settlement, consumption and crime. It can also force us to redefine fundamental concepts as work, family, life, and death.
At personal level, the experience and impact of new technologies is mediated and moderated by personal resources and constraints, such as skills and material resources (safety net). Subjective factors that also shape the individual or group response, like the general outlook (active or passive, optimistic or pessimistic, assertive or submissive, future- or past-oriented) can be inferred from observable consequences / circumstances, like experience of a technology-rich environment (see below), meaning the existence of a long-term pattern of the individual encountering and dominating technology.
More directly, the impact of new technologies, and AI in particular, can be seen in forms like :
- Job polarization (decrease in number and incomes of middle-skill, middle-wage, routine occupations jobs, and widening inequalities between those who have access to good-quality and skills-intensive work and those who end up being low-paid employees in inferior jobs)
- Technological unemployment (societal inability to invent new jobs faster than technology is destroying the old ones)
- Task automation and human replacement / Change in labor status due to automation
- Job quality (working time and work-life balance, job security, flexibility, autonomy and control over schedule, task variety and complexity).
Experience of a technology-rich environment, as mediating and moderating factor, can be measured by specific experiences, of by possession / use of specific items or services
- In daily life : self-driving car, smart & connected appliances, household digital hub, robotic child / elder care
- At work : regular human-machine interaction : with smart robots (design/development, supervision, training, service, collaborative robots) or AI (in medicine, services, administration), new occupations (robot coach, image labeller, algorithmization and automation of non-routine cognitive jobs)
All these can be measured by business and citizen surveys; Big Data and mobile apps could be used to investigate e.g. mobility and sociability patterns, or time use.
Of course, this is just a general sketch; concept refinement and operationalization is necessary before actual work can begin.
To sum up, measuring and monitoring the societal impact of AI is not going to be easy, fast, or cheap. We should aim, as well acknowledged by the HLEG request, to produce a multidimensional picture, where numbers are complemented by narratives illuminating the mechanisms of influence - which means, among other things, that a synthetic indicator or even a scoreboard, although attractive for policymakers, would be rather myopic and simplistic.
The key data collection methods, as already mentioned by other AI Alliance members, would be : an administrative AI census, business and citizen surveys, Big Data, web scraping and document collection. To all these I would suggest adding a funding line for small-scale, specialized research : ethnographic and organizational studies, psychological and behavioral economics experiments, medical and neurological investigations, econometric modeling.
I hope the information coming out of this project will acquire the same importance as macroeconomic data or the statistics underlying the Social Pillar. The information delivered should inform concerted policy and political response at EU level, therefore the raw data should be of verifiable quality and sustainable (continuously available to institutions). As a consequence, we cannot rely on data generated spontaneously by businesses and general public, but the AI monitoring project should be included in the EU statistical programme, supported by adequate legislation and funding.
- Kirjaudu sisään, jotta voit julkaista kommentteja
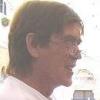
Fake news is the product of an asymmetry in the information process. The means of production and distribution overwhelm the means of digestion. The good, non-fake, news is that comparatively simple solutions are emerging from Explainable Artificial Intelligence that can flip that disadvantage into a collective win.
AI will effect all communities and activities. Commercial hype will realise expectations that cannot be met, and uninformed reaction will spread distrust and fear unless it is clearly understood and addressed. Fear of unrestrained AI has stalked the streets since "Blade Runner" hit the cinemas in 1982, and now even has a counter term "AI-Boxing".
However, understanding that reaction, especially once it is amplified via social media, presents real technical challenges. Supervised learning is inappropriate for discovery, and neural nets provide no explanation. Moreover it is clearly understood that a solution must also provide explainability and traceability, providing an obvious path from data to output. That suggests a reduction process.
J. R. Firth, was an English linguist and a leading figure in British linguistics during the 1950s. He first suggested the value of collocational analysis of texts, where word sequences are counted, such as 'strong tea'. As computers memories and environment have expanded the analysis has extended to skip-grams, where words need not be in a continuous sequence, they could just be in the same document. This accords with Firth's most well known maxim.
"You shall know a word by the company it keeps".
According to the Oxford English Dictionary just 100 words, such as pronouns and prepositions, account for half of the English corpus, carry little meaning, and can be ignored. Once such words are removed logically sound methods can generate all skip-grams present in the texts, and rank the association rules that such sets may suggest.
My own independent work has applied this technique on many document sets on many subjects,
and in many languages;
the skip-grams that emerge from this process represent the common ideas in the texts, combinations of people, places, and things, noun phrases.
Quite simply because I know of no other such analysis I'm struggling for the terminology. I think it exposes what people are talking about, and that it would therefore merit consideration as a mechanism for understanding feedback, helpfully in every EU language.
By chance eu4ai has just started a Twitter channel, so I can show you what I mean! Every 15 minutes a bot checks a Twitter list, spins out the stopwords, checks the combos, and stores the results ( because it gets really interesting to see how ideas change over time ). Here's the analysis, and you'll find more examples, academic papers and posters on the front page.
Enjoy!
- Kirjaudu sisään, jotta voit julkaista kommentteja