I am pleased to share with you the Report of our Research at Mozilla on AI Transparency in Practice.
Transparency is at the heart of responsible AI. In our study, we explore the concept of meaningful AI transparency, which aims to provide useful and actionable information tailored to the literacy and needs of specific stakeholders. We survey current approaches, assess their limitations, and chart out how meaningful transparency might be achieved.
Our study was conducted in light of upcoming regulation, such as the AI regulation in Europe's AI Act, the Federal Trade Commission’s increased attention to AI, and the US AI regulation on the horizon.
Based on surveys and interviews with 59 builders with transparency expertise from a range of organizations, the report examines the current state of AI transparency and the challenges it faces.
Findings include low motivation and incentives for transparency, low confidence in existing explainability tools, difficulties with providing meaningful information, and a lack of focus on social and environmental transparency. The report highlights the need for greater awareness of and emphasis on AI transparency, and provides practical guidance for effective transparency design.
In the absence of adequate ex-post explanation solutions, we encourage builders to consider using interpretable models rather than black-box solutions for applications in which traceability is a design requirement. We aim to build a community around best practices and solutions and raise awareness of transparency frameworks and methods.
Key Findings
- The focus of builders is primarily on system accuracy and debugging, rather than helping end users and impacted people understand algorithmic decisions.
- AI transparency is rarely prioritized by the leadership of respondents’ organizations, partly due to a lack of pressure to comply with the legislation.
- While there is active research around AI explainability (XAI) tools, there are fewer examples of effective deployment and use of such tools, and little conficence in their effectiveness.
- Apart from information on data bias, there is little work on sharing information on system design, metrics, or wider impacts on individuals and society. Builders generally do not employ criteria established for social and environmental transparency, nor do they consider unintended consequences.
- Providing appropriate explanations to various stakeholders poses a challenge for developers. There is a noticeable discrepancy between the information survey respondents currently provide and the information they would find useful and recommend.
Please don't hesitate to contact me for further discussions and research on AI Transparency. Our research continues!
Ramak Molavi Vasse'i
- Login for at skrive kommentarer
Kommentarer
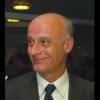
Thank you for sharing these results. That is helpful.
I would be pleased to get in touch for future joint work.
Best regards
Norbert Jastroch
- Login for at skrive kommentarer