“You can have your AI as soon as I have my gender-balanced society,” Margrethe Vestager, the Executive Vice President and Commissioner-Designate for Competition and Digital Policy, recently proclaimed before the European Parliament.
Vestager’s comments speak to concerns that artificial intelligence will perpetuate a further gender bias in society. From this perspective, algorithms reinforce discrimination when they produce results that differ significantly in accuracy across different demographic groups. Since machine learning systems often train their models on data that reflects real-world bias, many like Vestager are concerned that AI will perpetuate stereotypes.
While bias is a valid concern, is this the right response? Should Europe postpone the use of AI for decades until bias is eliminated, not just from the technology, but from society?
The answer is clearly no. In fact, AI can be a helpful tool for improving social fairness and gender equity in Europe.
First, AI can help identify and correct for human bias in society. For example, Disney recently started using an algorithmic tool to analyze scripts and track gender bias. Using a machine learning tool, the company could compare the number of male and female characters in scripts, as well as other indicators of diversity such as the number of speaking lines attributed to women, people of color, and people with disabilities.
In another example, companies are increasingly using AI in employee recruitment not just to speed up processes by screening and filtering the most relevant applications, but also to ensure decisions are not driven by unconscious biases by the human recruiters involved.
EU policymakers should also understand that it is not always a problem if algorithms perform differently across different demographics. For example, a women’s shoe store may use an algorithm that favours showing online ads to women over men to reduce its advertising costs.
Second, where algorithms generate biased results, policymakers should encourage the development of de-biasing tools that can make AI more impartial. For example, gender bias may appear in word embeddings—the association between two words, such as “woman” with “nurse” and “man” with “doctor.” Researchers have been able to effectively reduce gender bias in AI systems using different techniques, such as by resampling data. And many companies have developed open source tools to understand and reduce algorithmic bias, such as Microsoft Azure’s Face API, Facebook’s Fairness Flow, and Google’s What-If.
Including more women in the AI workforce would likely accelerate efforts to address gender bias and ensure women share in the benefits from AI. Ideally, companies should employ more diverse teams of developers and engineers to prevent cultural bias from entering systems inadvertently, and to ensure datasets do not include irrelevant or over- or under-represented elements. Unfortunately, companies adopting AI acknowledge that sourcing and retaining AI talent is a top challenge, and only 22 percent of the users on LinkedIn with AI skills are women—currently most AI experts are men.
EU policymakers should prioritize advancing digital skills among female students by integrating data science and computer science courses into school curriculums, particularly at the secondary school level, to inspire more women to gain interest in these fields. Policymakers should also foster an AI-friendly culture by initiating awareness-raising campaigns that articulate more clearly the value that AI and related digital technologies offer, and by encouraging educational institutions to organize more interaction between technology businesses and students. Making AI more attractive to women can expand the EU talent base and accelerate growth of the industry.
Finally, EU policymakers should update regulations that make it difficult for companies to gather enough data to improve their AI systems and innovate with solutions to tackle biases. Incomplete datasets can distort an AI system’s reasoning. Collecting useful data without fear of breaching rules will be critical to ensure algorithmic decision-making fosters social fairness. In addition, opening up the public sector’s data and expanding authorizations to use them can ensure companies have broader and safer access to higher-quality datasets. As one promising technique to tackle bias is the use of synthetic data to train algorithms on more diverse and reflective datasets, EU policymakers should encourage member states’ statistical agencies to release more of such data while protecting data privacy. They should also encourage the public and private sectors to collaborate on developing more inclusive data sets.
No technology is perfect, especially in its early stages of development. The job of policymakers is not to ban technology until it becomes perfect, for that is the path to technological stagnation. Rather, given the potential of AI and other emerging technologies both for societal and economic prosperity, policymakers should work to reduce harms while also enacting policies to improve their benefits and promote their long-term acceptance.
Building a fairer EU not only includes but depends on AI. This means working towards expanding diversity into teams creating algorithms, facilitating business access to more and richer data, adapting regulations to accelerate the adoption of AI, and, by increasing investment in AI, encouraging companies to develop methods that improve bias detection. Europe will not achieve greater social fairness and competitiveness in the digital economy by portraying technologies such as AI as inherently discriminatory. Instead, encouraging AI development will support efforts to reduce bias.
- Logáil isteach chun tráchtanna a phostáil
- Clibeanna
- AI Algorithmicbias womeninAI
Tráchtanna
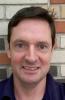
I really like this article. I am starting to run some experiments looking into gender bias and AI.
- Logáil isteach chun tráchtanna a phostáil
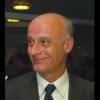
It may be wise to accept that AI will not be the answer to all questions which our societies face.
- Logáil isteach chun tráchtanna a phostáil

thank you for the article. I like the phrasing "AI can identify and correct human bias"
I would add "... quicker and with less conflict than with humans". so it lets human interact more like the ideal... unbiased and fair across the board.
- Logáil isteach chun tráchtanna a phostáil
In reply to thank you for the article. I by faiz ikramulla
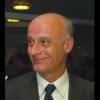
What you depict is 'streamlining', that is replacing the everyday social negotiation on micro-level between actors on social issues. In essence, this is rather the replacement of social processes by the execution of an automatted process which is based upon predefined selection criteria (what, by the way, is the perfect realization of "bias"). There is no good reason to expect this to lead to 'fair' results, but good reason to expect it to generate streamlined decision making by the exclusion of any variation (and variation, evolution theory tells us, is at the origin of evolution).
- Logáil isteach chun tráchtanna a phostáil
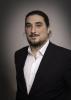
The issue we are dealing with in bias (be that gender, racial, based on income, etc.) in AI is that the data, the labels and most of the times the evaluation function are all created by humans and are inherently biased. We can see this clearly in risk assessment classification jobs for banks, where you take the data they have about people and whether loans were granted or not as the training for the algorithm. Using standard practices, the algorithm will always be biased if the data was biased. Similar problems will (and do) arise in recruitment through AI, and Disney`s "spellcheck".
This is not to say that AI can`t help. Machine Learning tools are wonderfully capable of finding out things that us humans are oblivious to or choose to ignore, and it can be quite enlightening to look at why an ML tool made the decision it made - and here is where I see the extreme value that AI can bring to combating bias in daily operations, as long as an overseer function is still a research topic instead of a tried and true method. Run the algorithms, and confront the decision-makers with the findings, let them agree (or disagree) with the results. Point out the bias in the training sets, and inherently in the whole process.
I would be interested in hearing more about your thoughts regarding how bias can be eliminated in other ways, as it is a challenge I do not yet see clear solutions for!
- Logáil isteach chun tráchtanna a phostáil
In reply to The issue we are dealing with by Marcell Ignéczi
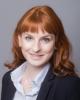
Hi Marcell,
Thank you for making the time to share your feedback on this.
Other solutions next to the one you've mentioned -- I know that education and awareness-raising are often mocked as solutions, because they're not a quick fix. But it definitely is one solid way to make changes in the long-term. And importantly, as mentioned in the op-ed, attracting and recruiting more women & diverse types of people into teams. There are many jobs in the field that don't necessarily require advanced digital skills. But ultimately, you also need more women trained in STEM, etc. So developers and engineers building algorithms will make sure to look at more possible issues getting into the system. The margin for improvement is quite big (80% of AI professors in prestigious U.S. universities are men, etc.), so that could mean it can only get better.
It's hard to debias data, there's no secret sauce. Even though you remove identifying variables like gender, proxy variables can still link back to gender. But correcting/adjusting is still something that should be done. Google did that with the "O Bik Doktor" issue you might have heard of.
Other solutions could be synthetic data: https://medium.com/solving-ai-bias-through-synthetic-data-facial/solving-bias-ai-through-synthetic-data-facial-generation-3911d5dd3986
I hope I've addressed your comment sufficiently and clearly enough, but let me know and again, many thanks for taking interest!
- Logáil isteach chun tráchtanna a phostáil

I had an interesting discussion this week with a colleague of mine.
We were looking for a use case where we could use AI and be compliant with ethical guidelines.
Since AI/ML tends to magnify existing biases in the data (if you in the past used to discriminate based on gender, then that discrimination will be in the data, and the algorithm will amplify that feature and it will enlarge the discrimination),...
Why not turn it around? Since you can actually use ML/AI to detect biases (making stereotypes bigger, is like putting a magnifying glass on it).
So now, you use your AI as a detection tool, an just like a negative feedback loop, you can now supress it (much like how an amplifier removes the noise).
It's making a feature out of a 'bug'.
- Logáil isteach chun tráchtanna a phostáil
In reply to I had an interesting by Christophe Cop
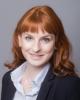
That's super interesting, thanks for sharing this example!
- Logáil isteach chun tráchtanna a phostáil