Dear members of the European AI Alliance,
As the Chair of the AI HLEG set out in his blogpost, the AI HLEG is looking for your contribution on the topics to be discussed at the First Workshop of the AI HLEG. These topics will also be addressed in the two main deliverables of the group.
The fifth topic of the workshop concerns: "Use Cases for the Guidelines"
This is the question on which your input is sought:
- Which Use-Cases are interesting/useful to explain, challenge and test the Guidelines?
Deadline for input: 13 September 2018
- Login for at skrive kommentarer
- Tags
- AI HLEG
Kommentarer
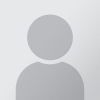
Hello all,
I would see the approach in the paper "Designing Human-Automation Interaction: a new level of Automation Taxonomy" as a helpful orientation for generating different use cases permutations.
Although the focus is on a classification of automating human-machine interactions, it can also be used for AI with small adaptations. "...The paper shows how these models are useful to understand the variable nature of automation support, but encounter limitations when identifying distinctive levels to analyse and compare concrete automation examples. Therefore existing automation levels are modified and adapted to the peculiarity of the four functions being confronted with current automation examples in support of both the flight crews’ and air traffic controllers’ activity..."
[[{"fid":"7547","view_mode":"default","fields":{"format":"default","field_file_image_alt_text[en][0][value]":"","field_file_image_title_text[en][0][value]":"The Level of Automation Taxonomy (LOAT)","field_caption[en][0][value]":""},"type":"media","attributes":{"title":"The Level of Automation Taxonomy (LOAT)","height":"664","width":"1103","class":"media-element file-default"}}]]
- Login for at skrive kommentarer
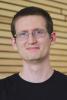
Use cases which tend to come up in fairness research regarding AI are systems which score and represent human beings, either as individuals or groups. For such systems, the guidelines should likely provide advice regarding the norms within which the systems should operate, both in terms of development and in terms of application. Examples include the following:
- Risk scoring for credit defaults, car crashes, or other kinds of financial risks. Insurance companies and banks are likely interested in obtaining accurate risk scores from diverse sources of data, and AI is likely to be applied to generate such risk scores. However, being assigned a high risk score is a financial burden to any individual. What are the guidelines in this case?
- Many on-line companies, e.g. in advertisement, are interested in representing their users according to certain groups, such as psychological profiles. AI systems can recognize such groupings or assign pre-defined ones. However, being assigned to a group can mean that private information is inferred without direct consent, groupings could reinforce stereotypes, and actions based on groupings could be discriminatory. What are the guidelines in this case?
- In search engines, AI systems are trained on natural language and on images in order to reveal typical human-like patterns and enhance search results. However, these kinds of adaptations to existing data can reinforce and even exacerbate existing biases and stereotypes, such as showing only white men when searching for pictures of 'CEO's, or associating women with work in the home while associating men with paid labor (Bolukbasi et al., 2016). What are the guidelines in this case?
- Public institutions may be increasingly inclined to guide decisions by AI systems in order to save resources. A prime example is predictive policing in the US. By analyzing statistical patterns in data of past crime, police patrols are guided to parts of the city where future crime is deemed more likely. This can result in overpolicing of regions with racial minorities and poor people, and underpolicing of regions with rich, white people (refer e.g. to O'Neil, 2016). What are the guidelines in this case?
I am aware that it is challenging to find a definitive response to any of these use cases, but I would be glad if the guidelines could raise awareness regarding the potential hazards of these kinds of AI systems.
- Login for at skrive kommentarer
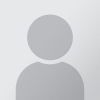
There are some key generic issues about AI Ethics. Some of these are about how do we want AI to work? Generally speaking we want it to be unbiassed, and explainable ( whatever that means in each case).
We must look at how whether these generic issues need to be managed differently in different use cases.
The two fastest emerging use cases for AI are Health and Transport. Both will require really high standards of explainability. Bias is likley to be more important in health than in transport.
- Login for at skrive kommentarer
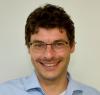
Possible Use Cases – Artificial Intelligence and Algorithmic Decision Making Processes
Explanatory remarks:
AI and Algorithmic Decision Making Processes (ADM processes) often raise similar Questions for policy makers. Particularly self-learning ADM proceses.
As soon as AI/ADM processes are based on personal data, privacy issues play an intrinsic role in these processes and their potential impact. Many of the risks in connection with AI/ADM processes mentioned below would increase if the level of data protection were lowered.
It is often postulated that data protection would prevent AI applications. It is overlooked that the GDPR already provides for far-reaching exceptions that also allow AI applications for personal data. For example, a change in the processing purpose is quite possible if the original purpose is compatible with the new/changed purpose. In addition, there are wide ranging exemptions for scientific research or statistical purposes - subject to safeguards - under which many AI applications can be included.
Moreover, data protection only plays a role at all when personal data are processed. However, this is not the case with many AI applications, such as the analysis of the weather or technical data of industrial plants (keyword: smart Factories).
Recommendation systems and decision assistants: Preserving the decision-making autonomy of individuals
- Examples: Smart Digital Assistants for Purchasing, media selection, health consulting, financial consulting
What is the problem?
- The lack of transparency about how the recommendations leads to a problematic knowledge asymmetry (to the detriment of the consumers), which can be exacerbated by a low level of data protection. While consumers can no longer grasp the decision-making processes and data processing, companies are learning more and more about individuals and consumers in general. As a result, the manipulability of consumers increases. Companies can exploit knowledge over consumers’ preferences or weaknesses. Results can be individualistic targeting, e.g. to automatically arouse latent wishes and needs in consumers or to differentiate the prices of their products accordingly. There is a danger that digital assistance systems, via the construction of digital identities of consumers according to group preferences, will lead in the medium term to the de-individualization of the same consumer preferences.
- Also: It is becoming increasingly difficult for people to distinguish whether they are dealing with an interaction with a human being or with an AI artifact.
- Relevance for consumers:
- Decision autonomy of individuals, self-determination, de-individualization, knowledge asymmetry, freedom of choice, market power/bottle neck
Possible approaches:
- Labelling obligation for AI systems that interact with consumers, or make decisions over consumers, establishment of a control system. This could entail transparency towards consumers and experts (audit team), meaningful explanation of decision logic including criteria and database.
Risk scoring and categorization of consumers: Determination of access to services and markets
Examples:
- Behaviour-based insurance tariffs, credit scoring, automatic job-candidate pre-selection, trustworthiness scores.
What is the problem?
- Many companies (e.g. financial service providers, traders, advertisers, platforms) use ADM and AI systems to create profiles of people and categorize them according to certain criteria into (fine-tuned) groups (e.g. financial performance, psychological profiles, socio-economic categories) in order to treat them differently.
- Service providers and platforms create trustworthiness scores of consumers with Machine Learing to enable transactions between unknowns.
- The most common machine learning methods are based on stochastic models. These use correlations between variables for pattern recognition and categorization. The resulting evidence is therefore not unambiguous (causal) but subject to uncertainties and error rates. These are inherent in the risk of a wrong decision or discrimination.
- Critical: Evaluation of data that have no relation at all to the target variable, but are used for the assessment via correlation models.
Relevance for consumers:
- Risk: Refusal to access certain markets and services (e.g. labor market, online trade, sharing economy, financial services, like unjustified refusal of credit or sole acceptance of prepayment in online shopping in the case of a negative score). There is a high financial damage potential, since unjustifiably high risk premiums could be demanded (interest on loans, higher rates for insurance tariffs).
- Protection against discrimination (also beyond sensitive groups "protected" in by law): The assignment to a group can mean that existing stereo types, socio-economic inequalities are reinforced (example: consideration of socially weaker job applicants who have longer travel times to the company and are therefore sorted out).
- Such problems weigh increasingly more heavily, since human intervention is often no longer possible. Once such analyses have made come to an assessment of a person, it may in future be very difficult for the person concerned to oppose this assessment and intervene in the event of negative decisions. To what extent will people in future be judged on the basis of their actual actions and not on the basis of predictions derived from their data (which could be unjustified)? What effect does it have if consumers only want to disclose their data very restrictively for reasons of data protection? Do these consumers have disadvantages because no data is available?
Possible Approaches:
- Duty to labelling, when decisions on consumers are based on scores. Transparency of the AI/ADM processes for consumers and experts (audit) with respect to decision logic, criteria and database, establishment of an independent control system, liability in case of damage. Possibilities to intervene in the case of unjustified decisions.
Smart Home: Ensuring liability
Examples:
- Smart entertainment products, home automation, smart meters, ...
What is the problem?
- AI applications, self-learning ADM-Process (even allegedly "simple" ADM processes) and are highly complex. This makes it virtually impossible for the consumer to prove, that a system had a “production”-fault in the event of damage (similar to medical "errors" done by doctors, e.g. during unsuccessful operations). In addition, a large number of service providers may be involved in the production process of the systems (codes and data could be integrated into the system from various providers). Likewise: Smart objects from different manufacturers increasingly combine to form a smart home network.
- This makes it difficult to clearly assign moral/legal responsibilities and liability in the event of damage. Example: Damage can be caused by malfunctioning devices (fictitious example: defrosting of the refrigerator, lawn mower hurting kids). Also: security gaps and data theft when manufacturers of networked devices in the smart home network have low security standards.
- This raises the question: Who is liable? How can a clear assignment of legal responsibility/liability for the case of damage result in complex AI or ADM systems? How can consumers be enabled to claim damages?
Possible Approaches:
- Liability in the event of damage caused by ADM and AI, or a low security Device in the network: Product liability regardless of fault lies with the manufacturer/supplier. Also for damages caused by connected devices. Result: The consumer has a contact person who is liable. The distribution of responsibility between the companies involved (within the internal relationship) is not the responsibility of the consumer (a task he is not up to anyway). Market mechanisms will lead to companies adopting high standards for security, not allowing their devices to connect to low-security devices.
Online trading: Preventing smart price cartels through the use of algorithms
What is the problem?
- It damages competition and consumers when competitors coordinate prices or conditions indirectly through algorithms. Not less when the coordinated behavior is achieved through self-learning algorithms.
- Conscious explicit parallel behavior or tacit collusion by self-learning algorithms can have a significantly higher damage potential compared to human-controlled parallel behavior.
Relevance for consumers and the economy
- In markets with high entry barriers, a small number of competitors and high market transparency, there is a risk that competition and innovation will be hurt and that consumers will pay higher prices. Cartels may be more stable since defection from cartel can be detected and sanctioned more quickly, by the cartel.
Posible Approaches:
- If the (self-learning) algorithms for price and condition setting are used to implement coordinated market behavior between, this must be sanctioned by competition authorities as an anticompetitive agreement. It must be clarified to what extent the companies that employ the algorithm can be held liable for tacit collusion ("coordinated" parallel behavior where the self-learning algorithms engage in parallel behavior without being implicitly being programmed to do so.)
Personalised prices: Power and knowledge asymmetries to the detriment of consumers
Examples:
Finely tuned price differentiation in online trading through collected user data. With the amount of information available about individual consumers, companies can manage an increasingly finely structured price differentiation (in real time). Apart from anecdotal evidence, there is currently no empirical evidence for the widespread use of personalized price differentiation. But the use of personalized discounts, both online and in food retailing, has economic equivalent effects.
What is the problem?
- Information and knowledge asymmetry, welfare losses for consumers/individual consumer groups
Relevance for consumers:
- Finely tuned (personalized) price differentiation can lead to welfare losses for affected consumer groups by having to pay higher prices than before.
- At the same time, it is by no means guaranteed that consumers with low payment power will open up new consumption opportunities through particularly low prices. In this way, possible positive welfare effects of price differentiation for consumers can be reversed and existing social fault lines reinforced.
Possible Approaches:
- An ethical discussion and decision are needed as to whether personalized prices should be banned in some social and economic sectors/contexts. The exploitation of health emergencies in terms of price is particularly questionable from an ethical point of view. E.g. by making health products (like special matrasses for people with back pain) more expensive for consumers who urgently need them.
- Data protection-conscious consumers must not be denied access to goods and services beyond what is legally required if they refuse to consent to data processing.
- Login for at skrive kommentarer