Dear members of the European AI Alliance,
As the Chair of the AI HLEG set out in his blogpost, the AI HLEG is looking for your contribution on the topics to be discussed at the First Workshop of the AI HLEG. These topics will also be addressed in the two main deliverables of the group.
The first topic of the workshop concerns: "Trusted (i.e. safe and responsible) AI"
These are the questions on which your input is sought:
- What does it mean to ensure trusted – i.e. responsible and safe – AI? Which tools can be implemented to achieve such trust?
- What does it mean to have trusted AI which supports and enables European businesses to be successful in applying AI? How can the above tools be implemented to achieve such businesses' success?
Deadline for input: 13 September 2018
- Login for at skrive kommentarer
- Tags
- AI HLEG
Kommentarer
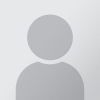
Arm supports the concept that AI should be ethical by design.
What does this mean:
(i) Every effort should be made to eliminate illegal and unfair bias in designing and developing AI decision systems.
(ii) We accept that some element of ‘bias’ is inevitable: algorithms designed for example to detect smugglers could be said to be ‘ biased’ in favour of detecting smuggling. But this is reasonable.
(iii) Algorithms in general are not unfairly biased. Training is the main problem. The careful use of training data is crucial in ensuring that illegal or unfair bias does not enter the AI system.
(iv) We recognize that this is not easy. More work needs to be done in this area.
(v) Careful scrutiny of new products and road maps should be the norm.
(iv) We also support the promotion of 'AI ethics' modules in training courses for AI engineers.
Arm also supports the concept of maximum useful transparency in explaining how AI works.
Arm believes that the tech sector should accelerate its efforts to provide ways in which AI machines can record and explain their decision making processes.
There are two distinct aspect of explainability. One is explaining how AI recognises patterns ( particularly images).We need more work at the technical level to clarify this.
The second is about how AI reaches its recommendations/decisions on human situations. Why did it turn down my mortgage application? This is what most commentators are worried about.
Arm supports the view that customers should be informed about how AI reaches its views/decisions.
But they need to be informed in a straightforward way. We need to avoid encouraging the proliferation of new Ts&Cs which no one reads.
What would ‘explainability’ look like? We think it could include:
- Explaining the role correlation plays in AI decision making and clarifying how this is not the same as causation.
- Explaining that AI decision making is probabilistic, not deterministic.
- Explaining, if possible, the key correlations/criteria used in reaching an AI decision.
- Explaining what would need to be different for the AI result to change ( eg if your salary was x dollars higher you would have got the loan)
- Explaining the use of filters/criteria (see above) to guard against unacceptable bias.
- Login for at skrive kommentarer
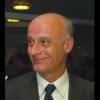
- Define "EU trusted AI application"
- Develop and publish a catalogue of criteria for it
- Implement a body that evaluates AI applications under these criteria
- Offer European and other businesses to undergo this evaluation and apply for the label "EU trusted AI application"
- Login for at skrive kommentarer
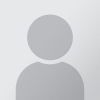
User account was deleted
- Login for at skrive kommentarer
Som svar til Trusted - i.e. responsible af Anonymous (ikke efterprøvet)
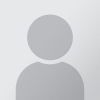
We can ensure the safety by keeping humans reponsible. If any AI driven device (robot or other) injures a human, the the owner and producer of that device share the responsibility.
I do not think we are seeking general humanity safety. I do not think there are signs of devloping something that should save humankid. We shold take care of us ourself. AI tool can help us with many things, but the responsibilôity remains with us.
I do not think that any global censorship is effective or even feasible. To manage thread we developed sofisticated legislative system that balces responsibilities, obligations and rights. An AI just another human product and humans must remain responsible for what they are doing.
- Login for at skrive kommentarer
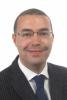
It means that the user of the AI system trusts its outcome. For instance, pilots trust decisions made by the autopilot in different flight phases and weather conditions. Likewise, radiologists should be able to trust diagnosis made by the AI system despite differences between patients. So I think a trusted AI system means that its outcomes are at least equivalent to the outcome achieved by a human expert or group of experts doing the same task in similar conditions.
An important point to note is that an AI system can be trustworthy under perfect conditions. However, it might become untrustworthy in case of uncertainties, uncertain measurements, and disturbances. So methods that model and analyze various forms of uncertainty (e.g. reachability analysis) can be used to evaluate the trust of the AI system. Evaluation can be performed against some defined targets (something similar to the target level of safety (TLS) measures used in aviation for safety verification and certification). Similar criteria can be used for autonomous systems in all modes of transportation (self-driving cars, automated ships, drones, etc.)
- Login for at skrive kommentarer
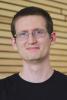
With respect to responsible and safe AI, it may also be worthwhile to talk about IT security aspects, namely whether AI aspects can be hacked and misused in novel ways. Examples in research include:
- Eykholt et al. (2018) have constructed traffic signs which look normal to humans but are misclassified by common neural networks.
- Athalye et al. (2018) have shown empirically that many protections against hacks can be broken as well.
- The field of differential privacy is concerned with the question whether machine learning models which have been learned on sensitive data may leak that data. While mostly theoretical, these approaches are now beginning to be applied in applications as well. Ji et al (2014) have provided an overview.
- In general, joining forces between classic IT Security research and AI may be promising to make AI systems more secure. One promising recent result is from Kilbertus et al. (2018), who developed a protocol to learn a fair machine learning model under encryption.
- Login for at skrive kommentarer

Trusted AI
Note : For the purpose of the present intervention, AI is considered primarily an automated decision system (ADS). The operator is the party deploying the AI/ADS.
Trust in ADSs can be gained by specific measures, such as those presented below.
Value-compliance : The set of principles underlying the functioning of any socially impactful AI/ADS should incorporate and embody fairness and justice. This could mean that the system has some necessary bias built in - e.g., the relaxation of economic or logistical criteria for optimization when they conflict with protection of vulnerable persons and of the environment. A related question : Is the algorithms' role to correct discrimination and injustice?
More generally, we must be mindful about the values reflected in the design and operation of AI/ADS, about what we choose to optimize and automate. We should also acknowledge that apparently neutral data reflect historical or systemic inequality and discrimination.
Values can enter an AI/ADS as as rules (e.g. in the justice system the preference is to minimize either false positives - no one wrongfully convicted - or false negatives - no one guilty acquitted; in the design and delivery of public services the preference is either to minimize public expenditures or to maximize coverage) and/or constraints (optimize transportation network BUT minimize environmental impact; optimize human resources BUT make sure no single mothers are disproportionately fired).
Value-compliance could be enacted as a checklist of pre-defined, mandatory societal values, to be filled in by the developer with the approach implemented to ensure compliance, and by the operator with the respective verifications. Preferably, the values included in the checklist are supplemented by their operationalization (see examples above), or should at least be operationalizable.
Opt-out : possibility to be excluded from any data operations and automated decision-making systems and processes, explicitly stated, and implemented as a straightforward, easy, trouble-free, and inexpensive procedure.
Challenge and reparations : automated decision can be challenged; the operator has the obligation to respond to the concerns of people who feel they have been incorrectly or unfairly assessed, and to rectify any harmful outcomes.
There are also two more complex components of trust, namely transparency and accountability; together, they form the foundation for a meaningful implementation of the right to know and challenge automated decisions. Their respective operationalization and implementation is presented further.
Transparency
Disclosure to the public and regulators : The operator should provide a definition of AI/ADS understandable by the general public. It should also explain the purpose of deploying AI/ADS, and (preferably) make explicit the expected performance of the system and the underlying assumptions about its operation.
The operator should disclose their current use of AI/ADS, accompanied by any related self-assessments and outside review processes and their respective results; for proposed use, the disclosure should ideally occur before a system has been acquired. The information should be detailed by types and classes of tools and processes, as well as areas of application (e.g., administrative; medical; with large-scale and long-lasting public impact - urban planning; impacting rights and entitlements - criminal risk assessment, employment, compensation) in order to facilitate review.
Use of an AI/ADS should be clearly indicated on any document (including web pages) that communicates the decision (or any output of the decision-making process) to the affected person / institution / group. It could (should?) include elements to allow the unambiguous identification of the specific algorithm used and possibly the training data set.
AI/ADS description should allow the assessment of: Data (sources, quality, bias, prohibited info - race, ethnicity, sexual preferences, etc., or proxies) ; Correctness / appropriateness of mathematical / statistical approach ; Correct understanding of the subject matter ; Proper usage, i.e., in the contexts and for the purposes the system has been designed for.
Understandable design : The AI/ADS should be specifically designed to be reviewed. Key among the disclosed information should be the properties that matter to automated decision making (see below, "Explainability"). Technical standards should be developed to this effect.
Explainability : This is a key trust-inducing mechanism.The AI/ADS should be accompanied by a natural language explanation of the decision input, process, and output. Although output is the most relevant, the system can generate the right output for the wrong reasons, which means that continued delivery of error-free decisions is not ensured.
As mentioned in a paper on legal accountability of AI, "explanations are usually required to answer questions like these: What were the main factors in a decision? Would changing a certain factor have changed the decision? Why did two similar-looking cases lead to different decisions?"
Accountability
Accountability is the antithesis of the assumption that AI-based systems and decisions are correct and don’t need to be verified or audited, and the twin concept of "moral outsourcing - the removal of human agency and responsibility from the outcomes of AI/ADS".
I suggest that the responsibility for the consequences of using AI/ADS be assigned to the operator. One key obligation of the operator should be to rectify any harmful outcomes.
Human-in-the-loop : the decision to deploy an AI/ADS, as well as any automated decision of such a system, should link back to a specific human decision-maker.
Monitoring and Auditing : The AI/ADS should be regularly audited; the operator should develop meaningful review processes, subject to independent control, that ensure continuous validation and testing, in order to discover, measure, and track impacts over time. This could include the compilation of a record of decisions and the respective context, as well as error rates and magnitudes, available (anonymized) to outside examination. The review process should allow the assessment of procedural regularity, i.e., that the same process was applied in all cases (see above, "Explainability").
The review and testing processes should allow external experts to apply the AI/ADS on their own data sets and then collect and analyze the output. The systems should be tested under a variety of conditions (sample size, data quality and completeness/ gaps, different operationalizations of the same concepts), to make sure that it behaves as expected, and to identify the circumstances under which it doesn't perform satisfactorily. Non-restricted access of external experts is necessary in order to compensate for the fact that the potential bugs in the system cannot be reasonably expected to be identified in the development phase. In time, after significant experience is acquired, regulators could demand a set of mandatory tests before certification.
Algorithmic accountability : the author of an article on how (and how not) to fix AI proposes "the principle that an algorithmic system should employ a variety of controls to ensure the operator can verify it acts as intended, and identify harmful outcomes should they occur."
- Login for at skrive kommentarer
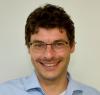
Consumers mistrust AI
AI and Algorithmic Decision Making Processes (ADM processes) often raise similar Questions for policy makers. Particularly self-learning ADM processes.
Uncertainty on AI currently prevails in the vast majority of society: Recent surveys show, that the vast majority of consumers perceives more risks than benefits when companies or government agencies make decisions automatically through algorithms. This isn’t really a surprise: Consumers have very little trust in Black-Box Systems. This mistrust also impedes the acceptance and pick-up of ADM and Artificial intelligence-driven Systems.
Consumers want information on the systems decision making and that the systems are controlled by an independent body. A representative survey by the vzbv from Dec. 2017 shows (https://www.vzbv.de/pressemitteilung/umfrage-verbraucher-wollen-kontrolle-ueber-ihre-daten):
i) Consumers want ADM processes to be labeled and info about the criteria (logic of the decision making)
ii) 75 percent regard automated decisions as a threat if the database and decision-making principles are unclear.
iii) 77 percent want auditability by the State
This tendency is confirmed by a recent survey of the Bertelsmann Foundation (https://algorithmenethik.de/2018/05/23/deutschland-noch-nicht-in-der-algorithmischen-welt-angekommen) as well as YouGov (https://www.telecompaper.com/news/germans-remain-sceptical-about-benefits-of-ai-technology-yougov--1260267)
How do consumers know, that the recommendadtions on products and services by smart digital assistants are in the best interest of the consumers? Example of the contrary: It is common practice that rankings on hotel booking platforms are determined by the provision that a hotel pays to the website (this info is hidden in the terms and conditions).
How can it be ensure that smart digital assistants like Alexa or Google Home act in the interest of the consumers and not in a corporate interest, when recommending products? This is particularly problematic as these companies become dominant market players and consumers are presented with only one recommendation (that they tend to select). Consumers can and do not simply switch to another assistant once it is established.
Making AI trustworthy: Transparency, Audits, Accountability
To realize the Chances of Artificial Intelligence theses system should be made trustworthy. Trust and acceptance could be promoted by two factors:
A) when automated decisions become transparent and explainable
b) when there is a proper control system in place, that makes sure that the decisions on consumers are lawful and ethically sound.
Therefore it should be considered to establish an independent control system that is able to review and audit socially relevant AI/ADM processes. Example credit scoring or the automatic selection of job applicants.
This audit should test whether the system conforms with legal laws: anti-discrimination law, unfair competition, data protection and it should analyse individual and social impact of AI.
In order to facilitate an audit in the first place we should consider establishing Standards for transparency-by-design and accountability-by-design. These could ensure that third party experts to get access to meaningful information. (e.g. Standards for documentation, APIs to test whether the data base is biased)
The focus should be on socially relevant ADM/AI processes. So those that potentially affect many consumers or have large adverse effects on them.
It should be considered to introduce proper information rights, labeling and publication obligations:
i) Consumers must be informed if decision is made automatically,
ii) Consumers must be informed about the main criteria and decision logic for the decision.
iii) Then consumers may object and correct the database.
iv) also for ADM-Processes using non-personal Data outside the GDPR, as many AI system do not rely on personal data)
IN sum: In order to establish trust, we need to ensure that these Black-Box Systems are independently controlled and audited, so that they adhere to the law.
72
Normal
0
21
false
false
false
DE
X-NONE
X-NONE
/* Style Definitions */
table.MsoNormalTable
{mso-style-name:"Normale Tabelle";
mso-tstyle-rowband-size:0;
mso-tstyle-colband-size:0;
mso-style-noshow:yes;
mso-style-priority:99;
mso-style-parent:"";
mso-padding-alt:0cm 5.4pt 0cm 5.4pt;
mso-para-margin:0cm;
mso-para-margin-bottom:.0001pt;
mso-pagination:widow-orphan;
font-size:10.0pt;
font-family:"Cambria",serif;}
- Login for at skrive kommentarer
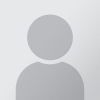
One way to achieve trust in an AI system, is by using interpretability (or explainable) AI. When designing such systems, it is important that the people involved at all levels (developers, stakeholders, users, policy makers) are satisfied with their understanding of how the solution works.
This is complex because different people have different needs for information. Typically users are given explanations in natural language or visual cues, that give information about the why of the prediction outcome. The following link explains this a bit better https://christophm.github.io/interpretable-ml-book/explanation.html
Developers want to know more. Typically the explanations given to the user won't help the developers correct the machine learning model, or understand when the model will misbehave, or what exact data records are causing the model to underperform. This complexity comes from the fact that every data record used to train the model will affect the prediction of the outcomes somehow. There are several tools available to help the developers:
- https://christophm.github.io/interpretable-ml-book/index.html
Several libraries and models that help you produce explainable models - https://ai.googleblog.com/2018/09/the-what-if-tool-code-free-probing-of.html
Recent tool from Google that lets you answer questions like: How would changes to a datapoint affect my model’s prediction? Does it perform differently for various groups–for example, historically marginalized people? How diverse is the dataset I am testing my model on? - https://dsapp.uchicago.edu/aequitas/ Open source libraries from the Center for Data Science and Public Policy of the University of Chicago.
An open source bias audit toolkit for machine learning developers, analysts, and policymakers to audit machine learning models for discrimination and bias, and make informed and equitable decisions around developing and deploying predictive risk-assessment tools. - DARPA's Explainable AI program https://www.darpa.mil/program/explainable-artificial-intelligence is pushing for techniques to:
- Produce more explainable models, while maintaining a high level of learning performance (prediction accuracy); and
- Enable human users to understand, appropriately trust, and effectively manage the emerging generation of artificially intelligent partners.
Here is a presentation with an onverview: https://www.cc.gatech.edu/~alanwags/DLAI2016/(Gunning)%20IJCAI-16%20DLAI%20WS.pdf - https://github.com/tensorflow/lucid A tool to peek into Neural network models.
- https://causalinference.gitlab.io/dowhy/ Causal inference should be a hard requirement for AI developers, to understand a bit more deeply what our models are really doing, and being able to simulate what-if scenarios to support decision makers better.
The field is exploding now with techniques on how to achieve this. But one should aim to produce Best Practices that people can align to. A forum where policy makers, Academia, and the industry can meet to discuss this would help pave the way to it.
There are lots of unsolved problems like:
- There is no formal definition of interpretability or explanation
- There is no objective measure of how interpretable a model is
- There are no experiments (as far as I know) about how much time it takes for a human to undertand an explanation
- How to audit explanations in an scalable way?
- How to design UX that facilitates the undertanding of an explainable system? F.ex. the following paper adresses this http://openaccess.city.ac.uk/13819/1/paper326.pdf
We should encorage the Industry and Academia to produce open research and open software, in a way that accelerates the efforts to get answers to the above problems.
- Login for at skrive kommentarer
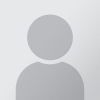
User account was deleted
- Login for at skrive kommentarer
Som svar til Great comments made below. af Anonymous (ikke efterprøvet)
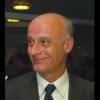
I couldn't agree more. This is what I meant when I suggested to develop 'criteria' for EU trusted AI applications.
A particular issue with this is the differentiation when it comes to specific applications: algorithmic software vs. artificial intelligence, automated vs. intelligent robotic operation, etc. .
Norbert Jastroch norbert.jastroch@metcommunications.de
- Login for at skrive kommentarer