Good morning and thanks for the opportunity to participate in such a critical conversation. One of the many aspects that I'd like to discuss with the group and have the HLEG weighting in is transparency in datasets characteristics and acquisition by AI startups.
As this forum is fully aware, a small and/or improperly gathered dataset can generate biased and/or inaccurate results. Both outcomes are not necessarily obvious until investigation but can have a very direct impact on consumers.
As part of my job, I interact with hundreds of technology startups per year, many of which, these days, claim to use modern artificial intelligence techniques to deliver what they promise. As part of my evaluation of these companies, I always ask how they acquired their dataset, what's its size, and what sort of analysis they performed to identify and mitigate a potential bias developed during the data gathering phase. I rarely receive clear, detailed, transparent answers, suggesting that this is a problem startups don't consider or care about, or that the dataset is not as data-rich as it should be.
Moreover, my interactions with data scientists on the subject suggest that very few are actually concerned about the risks of bias in datasets acquired directly or from 3rd parties or developed in a synthetic way.
My recommendation to end-user organizations interested in these AI startups always is to investigate how the companies acquire/build their datasets and what is their effort to evaluate both accuracy and bias. However, it would be in the interest of customers to have strict rules that force companies to disclose specific information about their datasets.
I'd be interested in hearing your opinion on the matter.
Thanks
Alessandro Perilli
GM, Management Strategy
Red Hat Inc.
@giano
linkedin.com/in/alessandroperilli
- Prisijunkite, kad galėtumėte skelbti komentarus.
Komentarai
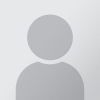
I think that additional transparency will definitely help improve public trust in AI especially if it covers not only start-ups but any company using algorithms. Still, there are a few issues that have to be taken into consideration and that may be difficult to solve:
1. Protection of intellectual property and commercial secrets - the more detailed the disclosure, the higher the risk that competitors will get access to the same dataset which may put smaller start-ups at a disadvantage vs larger and better funded companies
2. Impact on start-up activity - will dataset disclosure requirements push founders to move outside of the EU in the early years of their companies' existence?
3. Dataset exclusivity - should proprietary and third-party datasets be available to all interested parties similar to PSD2 in banking if this is needed to reduce/eliminate bias?
4. Disclosure level - should datasets be treated like opinion polls (disclosure of sample size and accuracy), gasoline (mandatory compliance with strict health and safety standards with room to use proprietary add-ons which significantly may impact performance) or food labels (an exhaustive list of all ingredients)?
One way to move forward maybe to introduce AI reporting similar to financial reporting which will include a) high level metrics that should be easily explained to the general population, b) additional notes that will give more assurance without disclosing sensitive information, and c) audit of algorithms and datasets by an independent third party (ideally not paid by the companies and not performed by the existing audit companies) which will be required for companies in sensitive industries and functions (e.g. health care, transportation, financial services; human resources) and voluntary for all other companies
In reply to I think that additional by Georgi Katanov
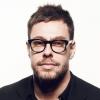
For now, I'll comment only on the first point:
There is no guarantee that access to the same dataset reduces competition. I represent a company that has built its reputation and fortune on open source software. Everybody has access to the same upstream code, which is freely accessible and transparent (just like AI datasets could be), and yet, startup companies remain quite competitive and able to thrive (to the point of being acquired in very remunerative exists).
The model works so well that today open source is the default choice for any ISV, no matter the size and funds.
I don't mean to turn this conversation into a conversation about the merits of the open source model. I am just using it as an example where access to the same primary resource doesn't impact competition as much as it would seem on paper.
That said, my proposal doesn't necessarily require to disclose the source of the dataset but at least some key characteristics, like the size and the methodology used to gather data.
In reply to For now, I'll comment only on by Alessandro Perilli
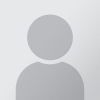
Red Hat is an outlier in the open source space and its success is at least partially the result of the value-added services for which it charges its clients. In the AI world we probably will end up with a similar set-up: most of the algorithms will be open source based on research papers or created and given away for free by the big tech companies and the differentiator will be the datasets that the companies use.
In reply to For now, I'll comment only on by Alessandro Perilli

I think you are right Alessandro, but you dont have to forget that algorithms and procedures to train neural nets (for example) still private choices. The better is the way you train neural nets the better is the result. More powerful computers (this is money ok?) less time to get results. If you have nice computers and high performance algorithms that gives you accuracy to get what are you searching you will have advantage.
So even if datasets are public, and the access is granted to all the world, results are different... do you agree ? im talking as software engineer.
So i think we need to talk about standards. This way an iA can be certified.