Dear members of the European AI Alliance,
As the Chair of the AI HLEG set out in his blogpost, the AI HLEG is looking for your contribution on the topics to be discussed at the First Workshop of the AI HLEG. These topics will also be addressed in the two main deliverables of the group.
The second topic of the workshop concerns: "Transparency and Accountability"
These are the questions on which your input is sought:
- What do the concepts transparency and accountability mean in an AI-context?
- How can their concrete and practical operationalization be ensured?
Deadline for input: 13 September 2018
- Illoggja biex tippowstja l-kummenti
- Tags
- AI HLEG
Kummenti
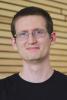
Thank you for asking for our input! Based on prior work in AI, I would suggest the following concepts for transparency and accountability.
Transparency:
- Besold et al. have put forward the notion of ultra-strong or comprehensible AI, referring to systems which can both express their learned hypotheses in symbolic form, and could teach a human observer how its internal reasoning works. In practice, this would require a system to provide a natural language explanation of the decision making process for any specific instance, of the hypotheses underlying the general decision making process, and these explanations have to be sufficiently concise to be understandable to a member of the general public without AI background. It is worth noting that such natural language explanations can not cover all details of the system, but rather give a rough overview.
- Christoph Molnar as recently released a book on interpretable machine learning, which suggests a host of statistical methods to gather insight into a system's decision making process. Examples include if-then explanations provided by decision trees, a list of features that have been used in the decision, and counterfactuals, that is, how the situation would have had to be different in order for the system to decide differently. These kinds of statistical explanations are likely not helpful to the general public, but are key to enable expert auditing.
- In its statement on algorithmic transparency and accountability, the ACM has several further suggestions regarding transparency, in particular: It should be made transparent on what kind of data the system has been trained and which kind of bias may be contained within that data. Ideally, the data itself should be available for further scrutiny, albeit in anonymized form such that privacy interests of citizens are taken into account. Further, the training process of any system should be recorded to be available for later auditing.
Regarding accountability:
- In line with the points made by Paul Nemitz in another thread, the ACM, and prior work of Joanna Bryson and others, I would suggest that legal liability for a system's actions and decisions should always rest with humans, and should not be pushed off to the unpredictability of the system itself. To but it bluntly: If a system went off its designated path so far that legal norms were severely violated, that's on the people who developed it and/or applied it. One question guiding such considerations may be: If a system was harmful, did its harm stem from its design as such or from its specific application context, e.g. biased training data, or over-interpretation of AI decisions? And if the latter is the case, did the developers of the system take sufficient measures to inform users of the system about these potential harms?
- In line with the GDPR, it would be useful to require any AI system which makes decisions over people to provide explanations for its decisions on multiple levels in the sense mentioned above, that is: A rough summary in natural language for the subjects of the decisions themselves, as well as more detailed information which can be used by experts as basis for potential legal measures.
- Finally, as recommended by the ACM, accountability should likely include regular audits of large-scale AI systems as well as continuous validation and testing measures, subject to independent control. If these safeguards suggest risk of harms, AI developers and/or users can be required to change the system.
- Illoggja biex tippowstja l-kummenti
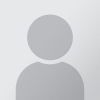
I would split the related measures, volulntary or enforced by law, into following areas:
1. Proper application of existing legislation, ethical and moral standards on AI
- as Benjamin Paaßen mentions in his response, usage of AI must not change the current setup of liabilites. Humans must remain responsible for the actions and decisions of AI, as they are with any other software systems or products now. The responsible humans must be able to explain the actions/decions of AI (with or without support of the system) as it is now with any other product.
- this implies, that compliance of AI with current legislation must be evaluated in broder context than other devices or tools. If anybody (vendor or user) wants to declare replacement of humans with AI, they must ensure compliance with all legislation and expectations as it would apply on human, including very basic and general ones, like moral and ethical acting, helping those in need, preventing damages, reporting unlawful activites, etc.Usage of AI must not create a gap in any existing regulations. E.g. if an AI driven tool is in a medical equipment where the user is expected to rely on the outputs, that equipment must pass all test as would any other such equipment AND its operator. If a doctor discriminating some patients would lose his license, so should any (AI driven) tool that may lead a honest doctor to discriminate some patients. If a human publisher would be punished for spreading false allegation, panic or so, so should be provider of an (AI driven) software tool or service selecting false allegation, panic or so for distribution to wider public. If AI is used in marketing, it cannot result in or be excuse for a prohibited marketing practice.
- This includes producer's responsibility to ensure that algorithms, training and validation sets are prepared with proper care to ensure compliance with law and regulations, including prevention of any biases or discrimination prohibited by law and to prove this on demand to respective government agencies (as likely as it is now with human activities)
- this includes no right of AI do defend itself by injuring a human, it can just report violence of human
- this is broad area and needs, above all, education of regulatory bodies, judges etc. about existence of AI, its legal status as an asset (no subjectivity to law), the human liabilty and that usage of AI is no excuse for any exemptions from the existing regulations
- just bacuse of some recent cases, this should include things like: ensuring equal availability of services and information, regardless of user's habits, behavior, psychological or other activities, or other characteristics - users must have control over the "bubble" around them and rules that filter the information available to them
2. Labeling AI: Ensuring easy distinguishing between human and AI actions and right to be informed about selected characteristics of AI one is dealing with (similar to information obligations in GDPR), which includes
- Clear notification of users and other affected persons that a product (car, tool, chatbot, news selection, search, etc.) is driven by AI with
- This is probably the simplest think we can do - create transparency about where AI is used,
- Proper explanation of the objectives, reliability, limits, biases, etc. in layman, non-technical language.
- create transparency about for what purpose and with what limitations, so the user and others can understand the outputs and use them properly
- Prevent misleading of humans, animals or commonly used tools by simulating or copying of appearance or behavior, which may make them believe that they interact with real human or animal
- We do this with other products - it would be illegal to sell a drug that looks like a normal food. Notification itself is not enough. In critical situations, people do not read notices, they act based on very basic instincts and the producers must respect these instinct in design of their products. Any AI driven tool must be distinguishable from human on first sight of any portion of the AI device.
- Proper distinguishing of AI tools from humans is in the benefit of producers as well, as it might allow reduction of their responsibility, especially if the behavior of the AI tool can be predicted by humans.
- Prevent misleading of humans, animals or tools in belief that a human, intentionally or accidentally, being considered AI driven device.
- Whatever sign is used to distinguish AI driven tools (humanoids) Make the distinguishing signs in the form that makes impossible to use such label on humans, intentionally or accidentally, because that may lead to loss of human rights, regardless it is temporary.
- Proper education of general public by researchers, designers, producers and governments
3. Prevention of abuse where AI creates new areas with potentially huge hard-to-assess damages
- AI is entering into communication with humans (and probably other areas) in way unseen before and we should find ways how to regulate these. Communication between humans is well regulated by law: prohibition of cheating, manipulation and other takctics applies to humans and should apply to communication with AI. AI tools might incline to or discover correlations in human behaviours which, if done by humans, might be considered as unlawful. Because it is a correlation discovered by AI, it is not an excuse for (ab)using it. This might need, for example, enhancement of prohibited marketing practices.
4. Traceability of AI actions
- legal system depends to high extent on (autonatic) memorization of what happened. This is important in investigations and evaluation of responsibility.
- Producers and users of AI should be obliged to monitor activities of their system and their compliance with law, regulation and ethical standards, especially if the algorithm is not deterministic. In case of any deviation that causes or might cause a breach of questionable activity, the producer and user must cooperate on appropriate remediation action, up to switching the system off.
- Saving and preserving of design and making it available in case of investigation
- This includes training sets, validation sets, models of neural network and coefficients/parameters used in the production environment/consumer products, decision trees, etc. so they can be investigated in case of damage caused by the product
- Saving and keeping logs of decisions and their reasoning at least as it would be expected from a human and making it available in case of investigation
- E.g. autonomous car must keep at least inputs from all sensors, log of recognized objects, log of all commands to all actuators as well as reasons for those commands - thinks corresponding to what is expected from human driver during investigation of any accident
- Ability to explain the outputs, including recommendations, decisions and actions, as already mentioned several times
- Illoggja biex tippowstja l-kummenti

Transparency and Accountability
Note : For the purpose of the present intervention, AI is considered primarily an automated decision system (ADS). The operator is the party deploying the AI/ADS.
Transparency and accountability are key components of public trust-building; together, they form the foundation for a meaningful implementation of the right to know and challenge automated decisions. Their respective operationalization and implementation is presented further.
Transparency
Disclosure to the public and regulators : The operator should provide a definition of AI/ADS understandable by the general public. It should also explain the purpose of deploying AI/ADS, and (preferably) make explicit the expected performance of the system and the underlying assumptions about its operation.
The operator should disclose their current use of AI/ADS, accompanied by any related self-assessments and outside review processes and their respective results; for proposed use, the disclosure should ideally occur before a system has been acquired. The information should be detailed by types and classes of tools and processes, as well as areas of application (e.g., administrative; medical; with large-scale and long-lasting public impact - urban planning; impacting rights and entitlements - criminal risk assessment, employment, compensation) in order to facilitate review.
Use of an AI/ADS should be clearly indicated on any document (including web pages) that communicates the decision (or any output of the decision-making process) to the affected person / institution / group. It could (should?) include elements to allow the unambiguous identification of the specific algorithm used and possibly the training data set.
AI/ADS description should allow the assessment of: Data (sources, quality, bias, prohibited info - race, ethnicity, sexual preferences, etc., or proxies) ; Correctness / appropriateness of mathematical / statistical approach ; Correct understanding of the subject matter ; Proper usage, i.e., in the contexts and for the purposes the system has been designed for.
Understandable design : The AI/ADS should be specifically designed to be reviewed. Key among the disclosed information should be the properties that matter to automated decision making (see below, "Explainability"). Technical standards should be developed to this effect.
Explainability : This is a key trust-inducing mechanism.The AI/ADS should be accompanied by a natural language explanation of the decision input, process, and output. Although output is the most relevant, the system can generate the right output for the wrong reasons, which means that continued delivery of error-free decisions is not ensured.
As mentioned in a paper on legal accountability of AI, "explanations are usually required to answer questions like these: What were the main factors in a decision? Would changing a certain factor have changed the decision? Why did two similar-looking cases lead to different decisions?"
Accountability
Accountability is the antithesis of the assumption that AI-based systems and decisions are correct and don’t need to be verified or audited, and the twin concept of "moral outsourcing - the removal of human agency and responsibility from the outcomes of AI/ADS".
I suggest that the responsibility for the consequences of using AI/ADS be assigned to the operator. One key obligation of the operator should be to rectify any harmful outcomes.
Human-in-the-loop : the decision to deploy an AI/ADS, as well as any automated decision of such a system, should link back to a specific human decision-maker.
Monitoring and Auditing : The AI/ADS should be regularly audited; the operator should develop meaningful review processes, subject to independent control, that ensure continuous validation and testing, in order to discover, measure, and track impacts over time. This could include the compilation of a record of decisions and the respective context, as well as error rates and magnitudes, available (anonymized) to outside examination. The review process should allow the assessment of procedural regularity, i.e., that the same process was applied in all cases (see above, "Explainability").
The review and testing processes should allow external experts to apply the AI/ADS on their own data sets and then collect and analyze the output. The systems should be tested under a variety of conditions (sample size, data quality and completeness/ gaps, different operationalizations of the same concepts), to make sure that it behaves as expected, and to identify the circumstances under which it doesn't perform satisfactorily. Non-restricted access of external experts is necessary in order to compensate for the fact that the potential bugs in the system cannot be reasonably expected to be identified in the development phase. In time, after significant experience is acquired, regulators could demand a set of mandatory tests before certification.
Algorithmic accountability : the author of an article on how (and how not) to fix AI proposes "the principle that an algorithmic system should employ a variety of controls to ensure the operator can verify it acts as intended, and identify harmful outcomes should they occur."
- Illoggja biex tippowstja l-kummenti

On the strength of the 16th CEPEJ Newsletter (August 2018, Theme: justice systems of the future, predictive justice, artificial intelligence and e-justice), I would like to focus on the possible use of AI risk assessment tools (RAT) by the judiciary in EU Member States. I think that since CoE picked up the theme of predictive justice, EU will soon follow.
Such AI RATs could potentially be used to assist the judiciary with the decision-making process or with courtroom fact-finding. Justice has a special position in our societies. It empowers individuals to protect themselves against inequities, and it encompasses core human rights, such as the right to a fair trial, and the right to an effective remedy. Therefore, justice constitutes a core element of our democracy, and matters related to our democracy are matters that should concern every EU citizen.
When it comes to the architecture of an AI RAT, the first element that needs special attention is inclusion in the decision-making process: Discussions about an AI RAT’s design should not take place behind closed doors. Instead, it is important for public authorities, civil society organizations, academics, and citizens, in general, to be able to actively participate in the decision-making process about this architecture. Inclusion creates also a sense of accountability since when the meetings are not secret their discussions are open to scrutiny. Also, inclusion creates transparency and boosts trust, since when you can actively participate, pose questions, express opinions, and receive clarifications, you feel involved and have confidence in the decisions agreed upon.
Since justice is the symbol of fairness, accountability, and transparency, the AI RAT used for its service should be enforcing these principles as well. Therefore, the architecture of the AI RAT shall not be protected by trade secrets because this would interfere with the open discussion process, as described above.
In addition, attention needs to be paid to the classification of the risk categories of an AI RAT. Using terms like “high risk” to classify individuals’ scores on AI RATs can influence the decision-making process of the judiciary and lead to biased choices. Furthermore, the potential of an AI RAT to perpetuate bias might exist in its source code as well, and this is another concern that requires attention. The quality of the data is of utmost importance for this assessment because if the AI RAT model is trained on biased data, it will be biased as well.
The intelligibility of an AI RAT is important as well. Even though intelligibility is hard to achieve, especially with more accurate AI RATs, like neural nets, it is a matter that should be addressed since it is linked to issues of transparency and fairness. In short, if the AΙ RAT concludes that an individual constitutes a high-risk, and the reasoning behind this conclusion cannot be explained, then consequently the judiciary’s decision based on such conclusion is neither transparent nor fair. Just like intelligibility, the relation of fairness with accuracy is problematic, since maximizing accuracy and fairness at the same time appears to be hard to achieve
It is essential that EU shapes AI to its own purpose and values. The EU Charter ensures the appropriate legal framework for AI in the EU. Fundamental rights shall be centrally reflected in the future guidelines of this group. Only in this way, such guidelines will truly support innovation and economic growth and foster individual freedoms.
- Illoggja biex tippowstja l-kummenti
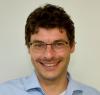
Some considerations of the vzbv on the issue of Transaprency and Accountability:
Consumers mistrust AI
AI and Algorithmic Decision Making Processes (ADM processes) often raise similar Questions for policy makers. Particularly self-learning ADM processes.
Uncertainty on AI currently prevails in the vast majority of society: Recent surveys show, that the vast majority of consumers perceives more risks than benefits when companies or government agencies make decisions automatically through algorithms. This isn’t really a surprise: Consumers have very little trust in Black-Box Systems. This mistrust also impedes the acceptance and pick-up of ADM and Artificial intelligence-driven Systems.
Consumers want information on the systems decision making and that the systems are controlled by an independent body. A representative survey by the vzbv from Dec. 2017 shows (https://www.vzbv.de/pressemitteilung/umfrage-verbraucher-wollen-kontrolle-ueber-ihre-daten):
i) Consumers want ADM processes to be labeled and info about the criteria (logic of the decision making)
ii) 75 percent regard automated decisions as a threat if the database and decision-making principles are unclear.
iii) 77 percent want auditability by the State
This tendency is confirmed by a recent survey of the Bertelsmann Foundation (https://algorithmenethik.de/2018/05/23/deutschland-noch-nicht-in-der-algorithmischen-welt-angekommen) as well as YouGov (https://www.telecompaper.com/news/germans-remain-sceptical-about-benefits-of-ai-technology-yougov--1260267)
How do consumers know, that the recommendadtions on products and services by smart digital assistants are in the best interest of the consumers? Example of the contrary: It is common practice that rankings on hotel booking platforms are determined by the provision that a hotel pays to the website (this info is hidden in the terms and conditions).
How can it be ensure that smart digital assistants like Alexa or Google Home act in the interest of the consumers and not in a corporate interest, when recommending products? This is particularly problematic as these companies become dominant market players and consumers are presented with only one recommendation (that they tend to select). Consumers can and do not simply switch to another assistant once it is established.
Making AI trustworthy: Transparency, Audits, Accountability
To realize the Chances of Artificial Intelligence theses system should be made trustworthy. Trust and acceptance could be promoted by two factors:
A) when automated decisions become transparent and explainable
b) when there is a proper control system in place, that makes sure that the decisions on consumers are lawful and ethically sound.
Therefore it should be considered to establish an independent control system that is able to review and audit socially relevant AI/ADM processes. Example credit scoring or the automatic selection of job applicants.
This audit should test whether the system conforms with legal laws: anti-discrimination law, unfair competition, data protection and it should analyse individual and social impact of AI.
In order to facilitate an audit in the first place we should consider establishing Standards for transparency-by-design and accountability-by-design. These could ensure that third party experts to get access to meaningful information. (e.g. Standards for documentation, APIs to test whether the data base is biased)
The focus should be on socially relevant ADM/AI processes. So those that potentially affect many consumers or have large adverse effects on them.
It should be considered to introduce proper information rights, labeling and publication obligations:
i) Consumers must be informed if decision is made automatically,
ii) Consumers must be informed about the main criteria and decision logic for the decision.
iii) Then consumers may object and correct the database.
iv) also for ADM-Processes using non-personal Data outside the GDPR, as many AI system do not rely on personal data)
IN sum: In order to establish trust, we need to ensure that these Black-Box Systems are independently controlled and audited, so that they adhere to the law.
vzbv proposes to discuss several measures that could feed into furthering transparency and accountability:
Review of relevant ADM/AI processes and case-specific measures
1. Inspection and assessment of legal conformity, individual and corporate impact
An appropriate control system, legitimized by the authorities should be able to inspect and verify relevant ADM/AI processes with regard to legal conformity (e.g. prohibition of discrimination, unfair competition law and data protection law), appropriateness of the application as well as individual and social effects. Whether an ADM/AI process should be inspected ex-post or ex-ante depends on the ADM/AI process in question and its area of application.
2. Determining the Relevance of ADM/AI Processes
It is necessary to develop relevance criteria to determine which ADM/AI processes warrant inspection. Where appropriate, further case-specific measures by a government-approved control system could be taken.
3. Determining the appropriateness of case-specific measures
Adequacy criteria must also be developed for deciding which case-specific measures should be taken to meet the challenges of specific ADM/AI processes. On this basis, it can be decided in each individual case which further measures should be taken. Examples of further measures can range from transparency requirements to the adaptation of the database or the algorithm to the implementation of the decision in the social context
a. Creating transparency for consumers and the public
There are ADM/AI processes that must be made transparent and comprehensible in order to enable sovereign consumer decisions and an informed public debate about the opportunities and risks of ADM/AI processes. Consumers should be informed about the use of relevant ADM/AI processes and about the relevant aspects of these processes (e.g. database, decision logic, target variables Y).
b. Adaptation of the ADM/AI process
The database, algorithm or other elements of the ADM/AI process must be designed in such a way that they themselves and the results of the ADM/AI process comply with legal requirements. If this is not the case, these components must be modified or withdrawn from circulation.
c. Ban as a last resort
A prohibition or legal prohibition of the use of certain ADM/AI processes can be a justified last resort in certain cases.
General Requirements for ADM/AI Processes
4. Ensure traceability: Traceability-by-design / explainability-by-Design / transparency by-Design
Rules and standards for the technical design of ADM/AI processes are required in order to meet legal requirements from the outset, to ensure ethics by design and to make ADM/AI processes accessible to control (e.g. Audit by experts).
5. Ensuring Falsification
Possible technical or methodological errors in ADM/AI processes must be made identifiable for suitable control systems (Audit done by Experts) and, if necessary, subjected to an independent scientific evaluation.
Need for action: Adaptation of the legal framework and social discourse on ethical principles
6. Create possibilities for challenging a decision
In ADM/AI processes that are not based on personal data, affected consumers should also have the right to have the decision reviewed by a person, to state their own position, to explain the decision and to contest the decision, e.g. in order to correct a false, distorted database or inappropriate decisions.
7. Introducing information rights, labelling and publication obligations
In order to satisfy the information needs of consumers on the use, decision-making processes, data basis and functioning of socially relevant ADM/AI processes, information rights, labelling and publication obligations must be introduced.
8. Adapting liability
Intransparency in ADM/AI processes and the increasing complexity of cause-and-effect chains can mean that consumers increasingly run risk to of not being compensated for damage caused by ADM/AI. Any gaps in liability in contract and tort law must be closed.
For the reform of the Product Liability Directive, liability for algorithms in the sense of genuine strict liability when used as intended by the consumer, independent of a fault, is an option. It should be sufficient for the provider's liability if an algorithm causes damage when used as intended.
v\:* {behavior:url(#default#VML);}
o\:* {behavior:url(#default#VML);}
w\:* {behavior:url(#default#VML);}
.shape {behavior:url(#default#VML);}
72
Normal
0
false
21
false
false
false
DE
X-NONE
X-NONE
/* Style Definitions */
table.MsoNormalTable
{mso-style-name:"Normale Tabelle";
mso-tstyle-rowband-size:0;
mso-tstyle-colband-size:0;
mso-style-noshow:yes;
mso-style-priority:99;
mso-style-parent:"";
mso-padding-alt:0cm 5.4pt 0cm 5.4pt;
mso-para-margin:0cm;
mso-para-margin-bottom:.0001pt;
mso-pagination:widow-orphan;
font-size:10.0pt;
font-family:"Cambria",serif;}
- Illoggja biex tippowstja l-kummenti
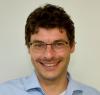
Consumers mistrust AI
AI and Algorithmic Decision Making Processes (ADM processes) often raise similar Questions for policy makers. Particularly self-learning ADM processes.
Uncertainty on AI currently prevails in the vast majority of society: Recent surveys show, that the vast majority of consumers perceives more risks than benefits when companies or government agencies make decisions automatically through algorithms. This isn’t really a surprise: Consumers have very little trust in Black-Box Systems. This mistrust also impedes the acceptance and pick-up of ADM and Artificial intelligence-driven Systems.
Consumers want information on the systems decision making and that the systems are controlled by an independent body. A representative survey by the vzbv from Dec. 2017 shows (https://www.vzbv.de/pressemitteilung/umfrage-verbraucher-wollen-kontrolle-ueber-ihre-daten):
i) Consumers want ADM processes to be labeled and info about the criteria (logic of the decision making)
ii) 75 percent regard automated decisions as a threat if the database and decision-making principles are unclear.
iii) 77 percent want auditability by the State
This tendency is confirmed by a recent survey of the Bertelsmann Foundation (https://algorithmenethik.de/2018/05/23/deutschland-noch-nicht-in-der-algorithmischen-welt-angekommen) as well as YouGov (https://www.telecompaper.com/news/germans-remain-sceptical-about-benefits-of-ai-technology-yougov--1260267)
How do consumers know, that the recommendadtions on products and services by smart digital assistants are in the best interest of the consumers? Example of the contrary: It is common practice that rankings on hotel booking platforms are determined by the provision that a hotel pays to the website (this info is hidden in the terms and conditions).
How can it be ensure that smart digital assistants like Alexa or Google Home act in the interest of the consumers and not in a corporate interest, when recommending products? This is particularly problematic as these companies become dominant market players and consumers are presented with only one recommendation (that they tend to select). Consumers can and do not simply switch to another assistant once it is established.
Making AI trustworthy: Transparency, Audits, Accountability
To realize the Chances of Artificial Intelligence theses system should be made trustworthy. Trust and acceptance could be promoted by two factors:
A) when automated decisions become transparent and explainable
b) when there is a proper control system in place, that makes sure that the decisions on consumers are lawful and ethically sound.
Therefore it should be considered to establish an independent control system that is able to review and audit socially relevant AI/ADM processes. Example credit scoring or the automatic selection of job applicants.
This audit should test whether the system conforms with legal laws: anti-discrimination law, unfair competition, data protection and it should analyse individual and social impact of AI.
In order to facilitate an audit in the first place we should consider establishing Standards for transparency-by-design and accountability-by-design. These could ensure that third party experts to get access to meaningful information. (e.g. Standards for documentation, APIs to test whether the data base is biased)
The focus should be on socially relevant ADM/AI processes. So those that potentially affect many consumers or have large adverse effects on them.
It should be considered to introduce proper information rights, labeling and publication obligations:
i) Consumers must be informed if decision is made automatically,
ii) Consumers must be informed about the main criteria and decision logic for the decision.
iii) Then consumers may object and correct the database.
iv) also for ADM-Processes using non-personal Data outside the GDPR, as many AI system do not rely on personal data)
IN sum: In order to establish trust, we need to ensure that these Black-Box Systems are independently controlled and audited, so that they adhere to the law.
vzbv proposes to discuss several measures that could feed into furthering transparency and accountability:
Review of relevant ADM/AI processes and case-specific measures
1. Inspection and assessment of legal conformity, individual and corporate impact
An appropriate control system, legitimized by the authorities should be able to inspect and verify relevant ADM/AI processes with regard to legal conformity (e.g. prohibition of discrimination, unfair competition law and data protection law), appropriateness of the application as well as individual and social effects. Whether an ADM/AI process should be inspected ex-post or ex-ante depends on the ADM/AI process in question and its area of application.
2. Determining the Relevance of ADM/AI Processes
It is necessary to develop relevance criteria to determine which ADM/AI processes warrant inspection. Where appropriate, further case-specific measures by a government-approved control system could be taken.
3. Determining the appropriateness of case-specific measures
Adequacy criteria must also be developed for deciding which case-specific measures should be taken to meet the challenges of specific ADM/AI processes. On this basis, it can be decided in each individual case which further measures should be taken. Examples of further measures can range from transparency requirements to the adaptation of the database or the algorithm to the implementation of the decision in the social context
a. Creating transparency for consumers and the public
There are ADM/AI processes that must be made transparent and comprehensible in order to enable sovereign consumer decisions and an informed public debate about the opportunities and risks of ADM/AI processes. Consumers should be informed about the use of relevant ADM/AI processes and about the relevant aspects of these processes (e.g. database, decision logic, target variables Y).
b. Adaptation of the ADM/AI process
The database, algorithm or other elements of the ADM/AI process must be designed in such a way that they themselves and the results of the ADM/AI process comply with legal requirements. If this is not the case, these components must be modified or withdrawn from circulation.
c. Ban as a last resort
A prohibition or legal prohibition of the use of certain ADM/AI processes can be a justified last resort in certain cases.
General Requirements for ADM/AI Processes
4. Ensure traceability: Traceability-by-design / explainability-by-Design / transparency by-Design
Rules and standards for the technical design of ADM/AI processes are required in order to meet legal requirements from the outset, to ensure ethics by design and to make ADM/AI processes accessible to control (e.g. Audit by experts).
5. Ensuring Falsification
Possible technical or methodological errors in ADM/AI processes must be made identifiable for suitable control systems (Audit done by Experts) and, if necessary, subjected to an independent scientific evaluation.
Need for action: Adaptation of the legal framework and social discourse on ethical principles
6. Create possibilities for challenging a decision
In ADM/AI processes that are not based on personal data, affected consumers should also have the right to have the decision reviewed by a person, to state their own position, to explain the decision and to contest the decision, e.g. in order to correct a false, distorted database or inappropriate decisions.
7. Introducing information rights, labelling and publication obligations
In order to satisfy the information needs of consumers on the use, decision-making processes, data basis and functioning of socially relevant ADM/AI processes, information rights, labelling and publication obligations must be introduced.
8. Adapting liability
Intransparency in ADM/AI processes and the increasing complexity of cause-and-effect chains can mean that consumers increasingly run risk to of not being compensated for damage caused by ADM/AI. Any gaps in liability in contract and tort law must be closed.
For the reform of the Product Liability Directive, liability for algorithms in the sense of genuine strict liability when used as intended by the consumer, independent of a fault, is an option. It should be sufficient for the provider's liability if an algorithm causes damage when used as intended.
v\:* {behavior:url(#default#VML);}
o\:* {behavior:url(#default#VML);}
w\:* {behavior:url(#default#VML);}
.shape {behavior:url(#default#VML);}
72
Normal
0
false
21
false
false
false
DE
X-NONE
X-NONE
/* Style Definitions */
table.MsoNormalTable
{mso-style-name:"Normale Tabelle";
mso-tstyle-rowband-size:0;
mso-tstyle-colband-size:0;
mso-style-noshow:yes;
mso-style-priority:99;
mso-style-parent:"";
mso-padding-alt:0cm 5.4pt 0cm 5.4pt;
mso-para-margin:0cm;
mso-para-margin-bottom:.0001pt;
mso-pagination:widow-orphan;
font-size:10.0pt;
font-family:"Cambria",serif;}
- Illoggja biex tippowstja l-kummenti
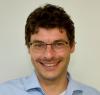
vzbv proposes to discuss several measures that could feed into furthering transparency and accountability:
Review of relevant ADM/AI processes and case-specific measures
- Inspection and assessment of legal conformity, individual and corporate impact
An appropriate control system, legitimized by the authorities should be able to inspect and verify relevant ADM/AI processes with regard to legal conformity (e.g. prohibition of discrimination, unfair competition law and data protection law), appropriateness of the application as well as individual and social effects. Whether an ADM/AI process should be inspected ex-post or ex-ante depends on the ADM/AI process in question and its area of application.
- Determining the Relevance of ADM/AI Processes
It is necessary to develop relevance criteria to determine which ADM/AI processes warrant inspection. Where appropriate, further case-specific measures by a government-approved control system could be taken.
- Determining the appropriateness of case-specific measures
Adequacy criteria must also be developed for deciding which case-specific measures should be taken to meet the challenges of specific ADM/AI processes. On this basis, it can be decided in each individual case which further measures should be taken. Examples of further measures can range from transparency requirements to the adaptation of the database or the algorithm to the implementation of the decision in the social context
- Creating transparency for consumers and the public
There are ADM/AI processes that must be made transparent and comprehensible in order to enable sovereign consumer decisions and an informed public debate about the opportunities and risks of ADM/AI processes. Consumers should be informed about the use of relevant ADM/AI processes and about the relevant aspects of these processes (e.g. database, decision logic, target variables Y).
- Adaptation of the ADM/AI process
The database, algorithm or other elements of the ADM/AI process must be designed in such a way that they themselves and the results of the ADM/AI process comply with legal requirements. If this is not the case, these components must be modified or withdrawn from circulation.
- Ban as a last resort
A prohibition or legal prohibition of the use of certain ADM/AI processes can be a justified last resort in certain cases.
General Requirements for ADM/AI Processes
- Ensure traceability: Traceability-by-design / explainability-by-Design / transparency by-Design
Rules and standards for the technical design of ADM/AI processes are required in order to meet legal requirements from the outset, to ensure ethics by design and to make ADM/AI processes accessible to control (e.g. Audit by experts).
- Ensuring Falsification
Possible technical or methodological errors in ADM/AI processes must be made identifiable for suitable control systems (Audit done by Experts) and, if necessary, subjected to an independent scientific evaluation.
Need for action: Adaptation of the legal framework and social discourse on ethical principles
- Create possibilities for challenging a decision
In ADM/AI processes that are not based on personal data, affected consumers should also have the right to have the decision reviewed by a person, to state their own position, to explain the decision and to contest the decision, e.g. in order to correct a false, distorted database or inappropriate decisions.
- Introducing information rights, labelling and publication obligations
In order to satisfy the information needs of consumers on the use, decision-making processes, data basis and functioning of socially relevant ADM/AI processes, information rights, labelling and publication obligations must be introduced.
- Adapting liability
Intransparency in ADM/AI processes and the increasing complexity of cause-and-effect chains can mean that consumers increasingly run risk to of not being compensated for damage caused by ADM/AI. Any gaps in liability in contract and tort law must be closed.
For the reform of the Product Liability Directive, liability for algorithms in the sense of genuine strict liability when used as intended by the consumer, independent of a fault, is an option. It should be sufficient for the provider's liability if an algorithm causes damage when used as intended.
- Illoggja biex tippowstja l-kummenti
In reply to vzbv proposes to discuss by Miika Blinn
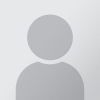
We should keep the amount of regulation under control. Too much regulation might limit further development in the area. I think that nearly all critical areas are already identified in existing legislation and it is more about its proper application. We have to think of small companies that experiment with new technology and bet their money and time to develop something new. Despite we have to prevent (or reasonably reduce) the wrongdoing, we should not make entering the market too difficult by extensive regulation. It is appropriate to keep customers informed about AI usage. It is also appropriate to expect documentation of the system available, including informing the customer about reliability of the decisions, as it is done during development anyway, however we should be realistic in how specific we are with these requirements.I would prefer rather limited specific AI regulation and prefer proper application of existing general rules. There are sensitive areas today, where the requirements are very specific, like medicine, and so should be with AI driven systems. There are areas where we have more freedom, because they are not so critical, like retail sales, where we should keep enough space for creativity.
- Illoggja biex tippowstja l-kummenti
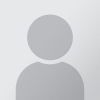
I hope you are still admiting feedback.
Transparency is characterized by the visibility or accessibility of information especially concerning all the processes involved in the implementation of the AI solution. This means that information about the following should be made publicly available:
- How the data was collected
- How the data is cleaned (the preprocessing step before feeding the data to a machine learning model) and encoded
- What kind of model is used (type, parameters, model architecture, hyperparameters)
- What kind of bias exploration has been done on the data
- What kind of measurements are done to make prevent the deployed AI solution from misbehaving (for example detect model drifting, or change in the distribution of the training data and the production data)
- What measures ensure that the AI solution is not leaking private information from the users, used at the training step (some people have already mentioned differential privacy)
Observe that there is no notion of making this explainable to the public. But the point of transparency is that experts can audit the whole process, and in the best case be able to reproduce the complete pipeline.
Accountability refers to the capability of being explained or held answerable. I align myself with the answer from https://ec.europa.eu/futurium/en/users/benjamin-paassen which cover the legal aspects of the answer, and doing regular audits on the AI solution.
Thinking practically on how to implent these principles operationally, one sees right away that:
- There should be clear guidelines (or standards) that help organizations comply to these principles. A good example is provided in the following link: https://cdt.org/issue/privacy-data/digital-decisions/
This interactive graphic is meant to bridge the gap between the principles described above and the practical realities of designing a product or service for a dynamic market. As you click on each step of creating an algorithm, the next phase of product development will open up. Ultimately, you will access a series of questions that are meant to provoke thoughtful consideration of potentially biased decisions that may lead to disparate outcomes. The questions can be addressed in whatever order makes sense for your project. There is not necessarily one right or wrong answer to any of these questions. They are intended to raise awareness of and help mitigate potential areas of unintended bias or unfairness.
- In my opinion, a neccesary requirement to implement audits for accountability is reproducibility. That is, one should be able to reproduce all the steps in a machine learning pipeline. For example, look at the following link https://machinelearningmastery.com/reproducible-machine-learning-results-by-default/
Technology like kubernetes, docker, kubeflow are examples of standards that help organizations build reproducible results. - Transparency requires also using transparent, explainable models when possible. Use of "black box" models for systems that take digital decisions is problematic due to their nonlinear nature. Even though one can produce an explanation for each prediction, it doesn't mean that one has control over all possible scenarios. A small change in one of the input features can give a big impact in the prediction outcome. That makes it difficult to reason about them. As pointet out by Benjamin Paaßen the book by Christoph Molnar gives good examples of transparent models. Otherwise the following article has a good survey: https://arxiv.org/abs/1802.01933
- Illoggja biex tippowstja l-kummenti